Josh Barua
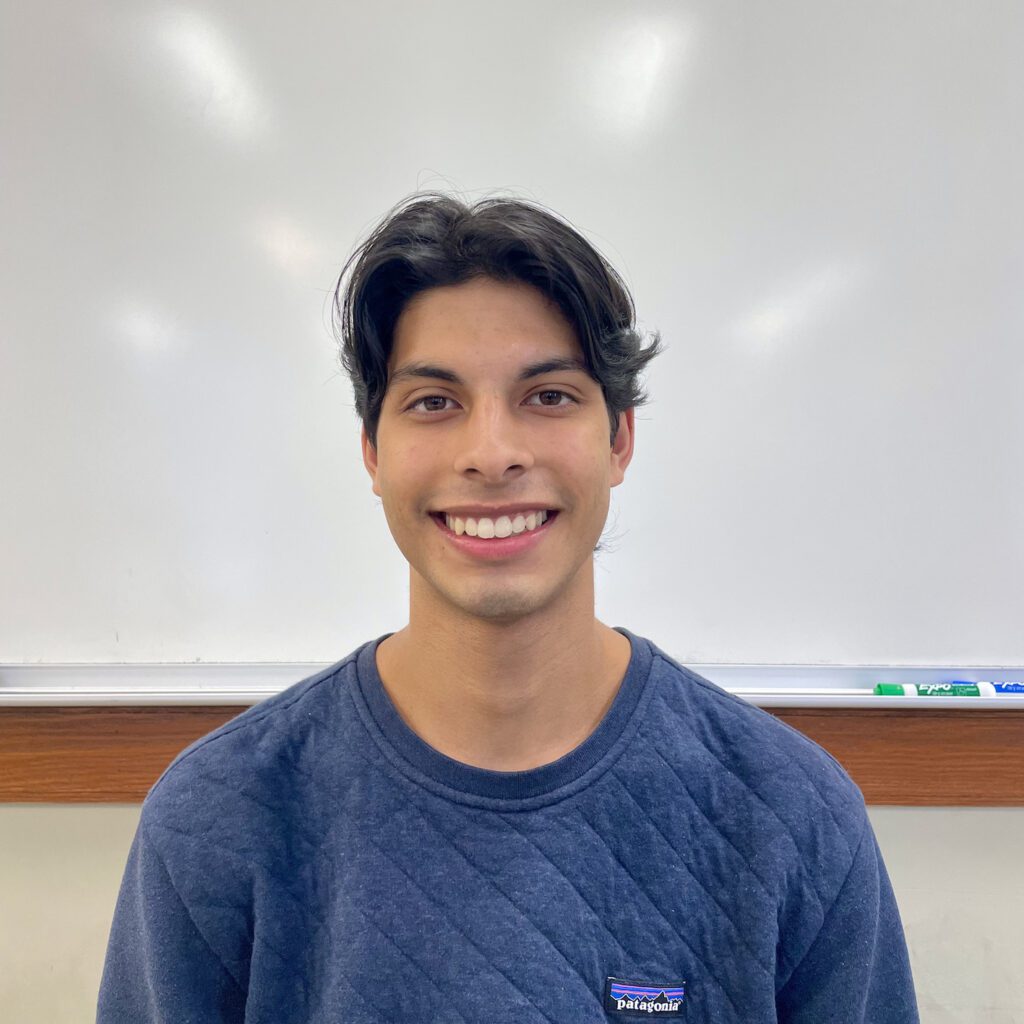
The emergence of unique semantic subdivisions of concepts across languages is a natural byproduct of cultural, geographic, and historical factors. One example of concept variation is the choice between “boli” and “pluma” when translating “pen” in Spanish. While both loosely translate to pen, boli typically refers to a ballpoint pen whereas pluma refers to a fountain pen (or even a quill in historical contexts). For non-native speakers, learning these subtle lexical rules that govern which translation to use can prove challenging without expert help. For my research project, we aim […]
Hong Joo Ryoo

As a SURF fellow, my research project focuses on testing the effectiveness of a proposal presented in a previous paper for quantum computations of scattering observables when three-body intermediate states are involved. The proposal offers a strategy to deal with the finite system size and periodic spatial volume limitations that current quantum computation strategies face. We aim to investigate the impact of boundary conditions on the extraction of hadronic and Compton-like scattering amplitudes and quantify the systematic uncertainty that arises. By applying the proposed improvement strategy based on the reduced […]
Raela Richie
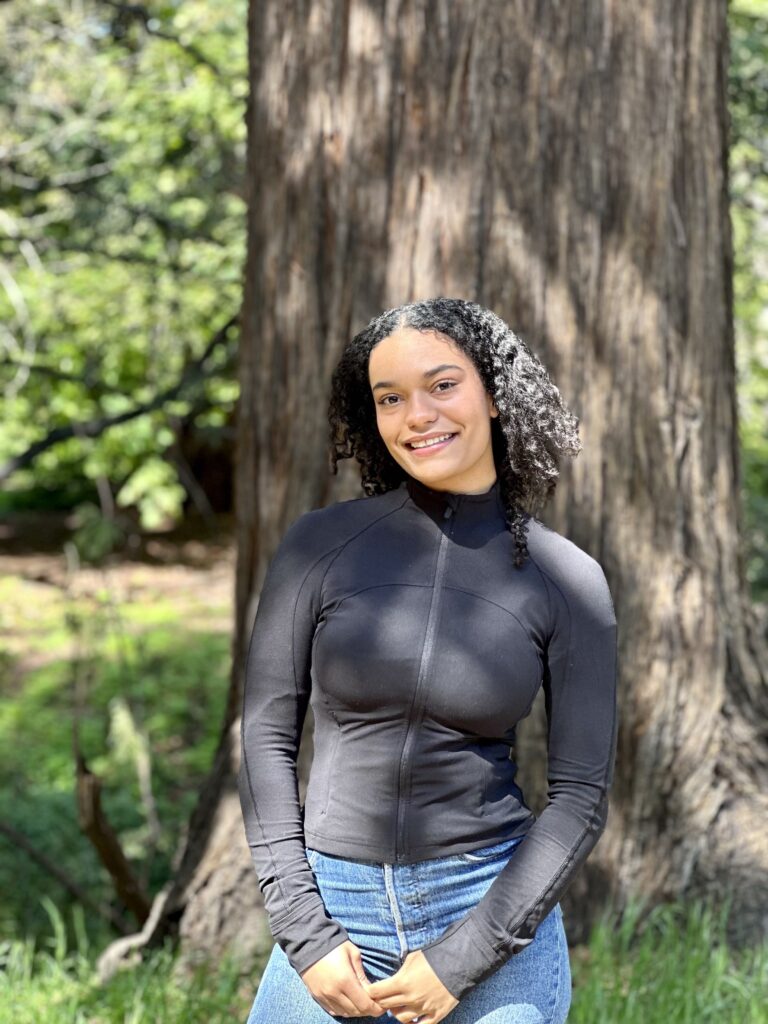
Kīlauea Volcano has exhibited both effusive and explosive behavior over several centuries, with recent studies suggesting that a 1790AD eruption that killed hundreds of native Hawaiians was not an isolated event. Despite the likelihood of future explosive eruptions, we do not understand what changes in the volcanic plumbing system or magma chemistry cause these shifts in eruptive style. This project will use the relationship between pressure and CO2 density in pockets of CO2-rich fluid trapped within growing crystals to constrain the depths of magma storage at Kīlauea during the last […]
Berenise Rangel
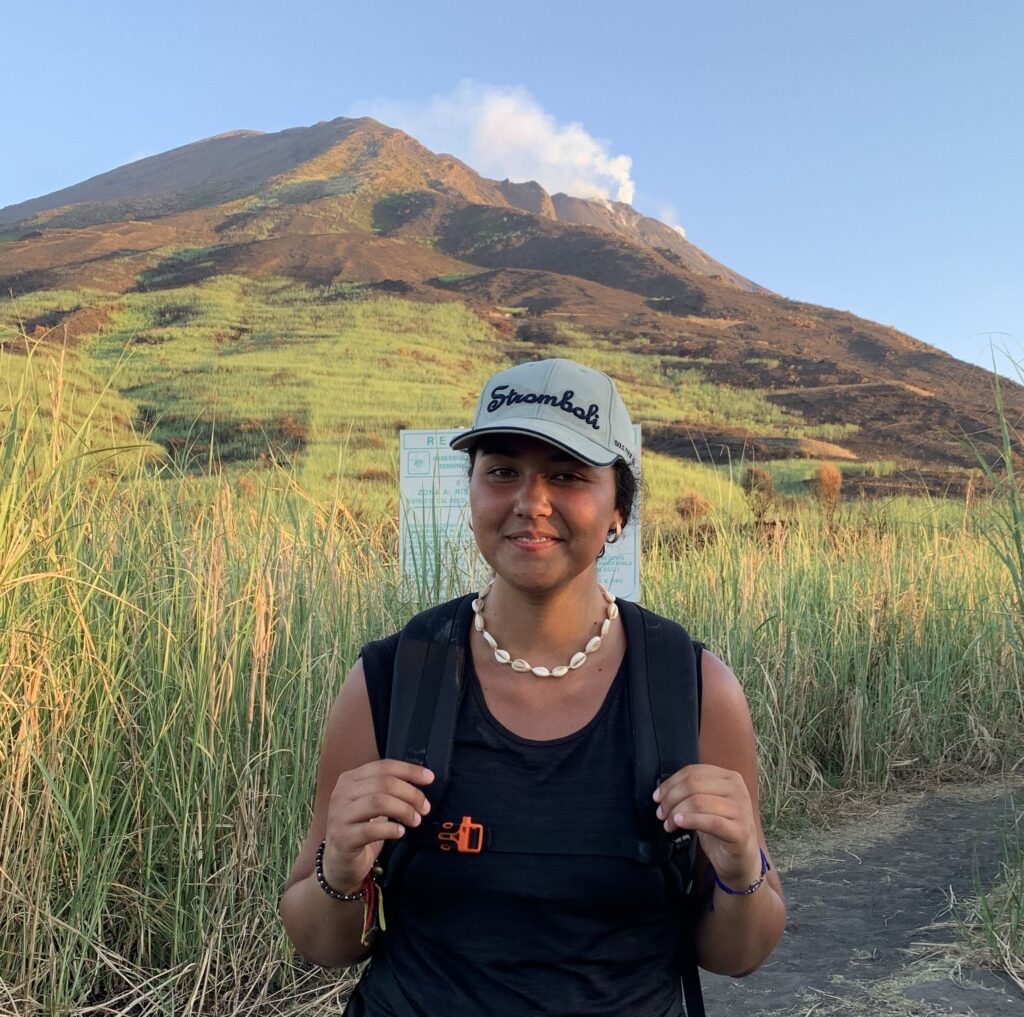
Volcanic eruptions present a major hazard to society, both in terms of infrastructure destruction through lava flows, and their influence on the Earth’s atmosphere and climate. Mauna Loa, HI, has the largest volume of any volcano on Earth, and is currently in its fastest growing ‘shield phase’ (meaning numerous lava flows have erupted over the last millenia, and many more are expected).Given the rapid growth and clear hazards presented by this volcano, it is absolutely vital to understand the magmatic plumbing system supplying lava to the surface. I will determine […]
Ellie Mak
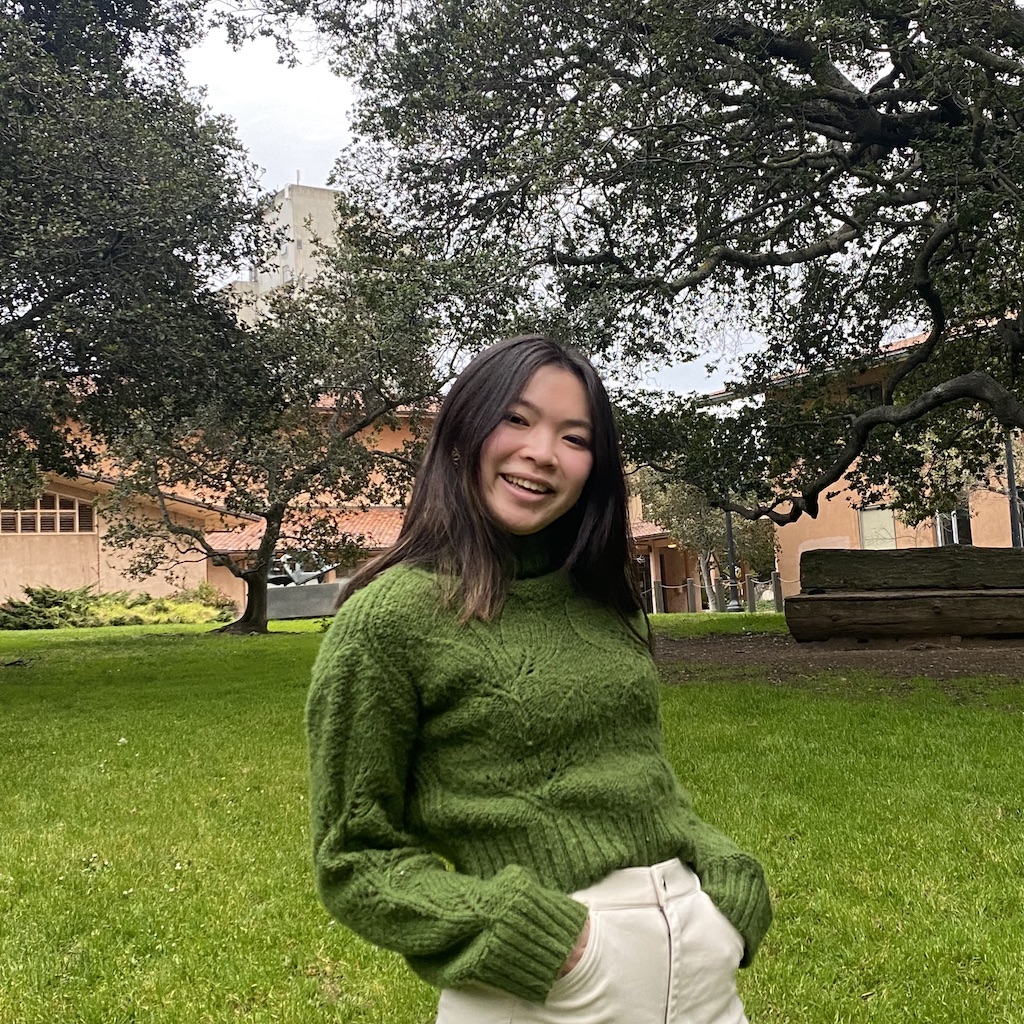
Approximately 85% of the Universe’s mass consists of an invisible non-atomic material called dark matter. It has shaped our Universe, including the formation and evolution of galaxies, and without it, humans would not exist. However, despite both its centrality in cosmology and decades of experimentation, the microscopic characteristics of dark matter particles are still unknown. This project focuses on detecting the products of possible dark matter self-annihilation in the Galaxy to reveal its origin and particle characteristics; however, the large and uncertain astrophysical backgrounds pose a challenge. GAPS is a […]
Ekansh Agrawal
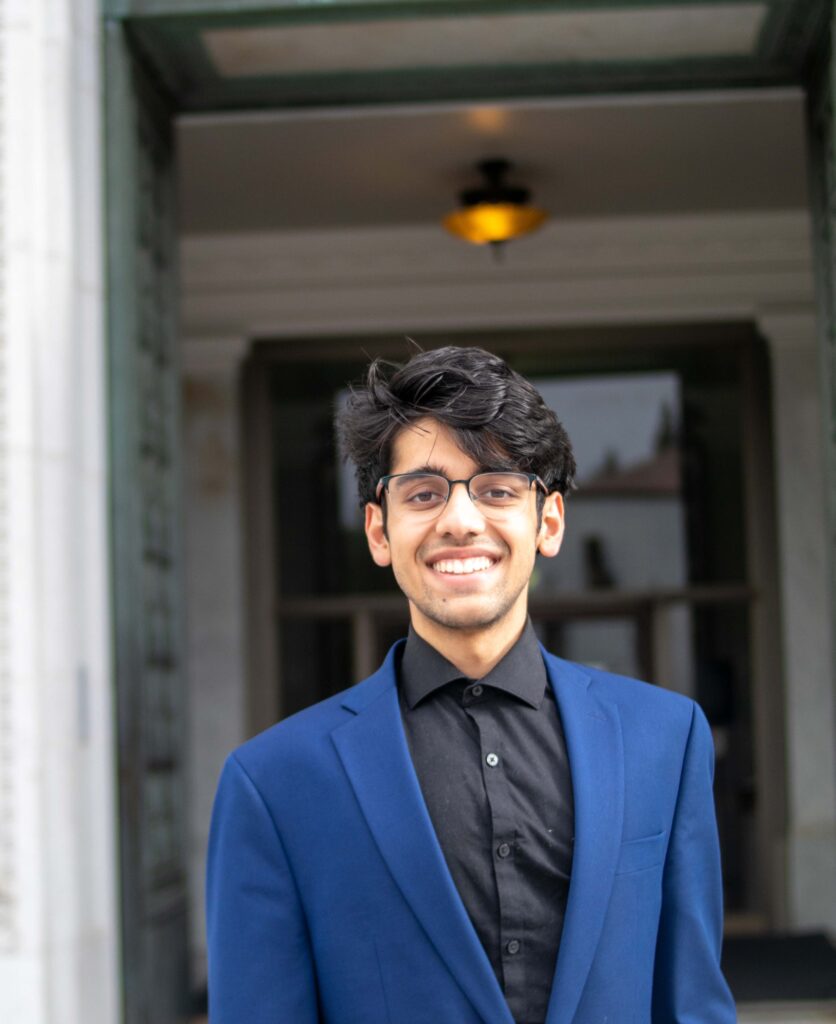
My research project seeks to delve into the transformative impact of social media on e-commerce over the past decade. With the widespread adoption of social media platforms, user engagement has skyrocketed, presenting a unique opportunity for businesses. Specifically, I aim to focus on TikTok, a platform with a staggering 700 million daily users, and examine the advertising landscape within this dynamic space. By studying sub-communities on TikTok and their response to content, I aim to understand the mechanisms that contribute to the concept of “virality” in the realm of e-commerce […]
Pablo Castaño Basurto
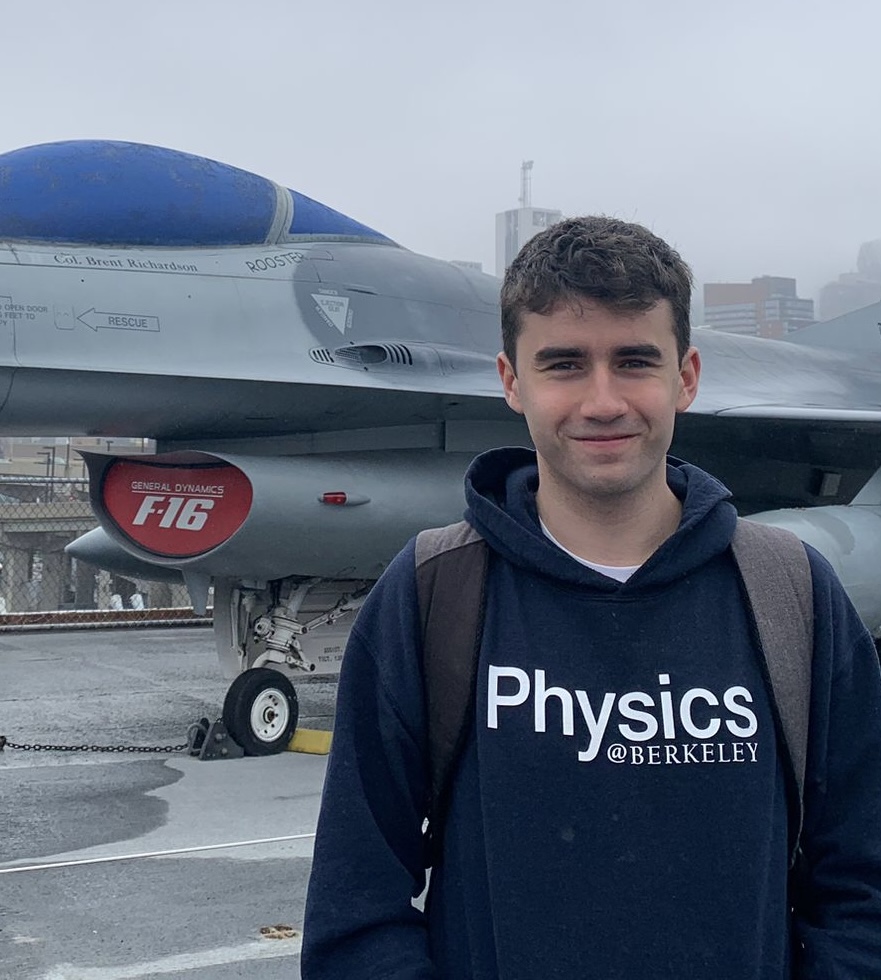
Physicists view the universe through the lens of two theories: Einstein’s theory of general relativity and quantum field theory (QFT). The former describes gravity as the curvature of spacetime due to the presence of matter and energy, while the latter tells us that elementary particles can be thought of as excitations of fundamental “fields”. However, these theories are not complete, and there are many questions about the fundamental nature of space, time, and our universe that remain unanswered. Chern-Simons gravity, a theoretical framework that describes the behavior of three-dimensional spacetime, […]
Matthew Bilotta
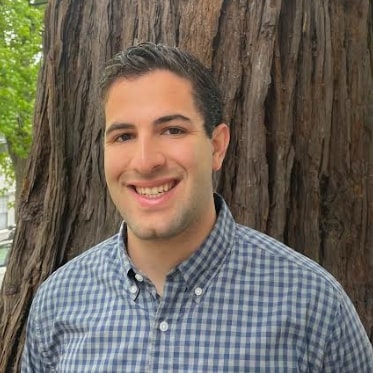
In modern atomic physics, it is necessary to have very stable frequencies of laser light. However, the stability of a laser will often deviate from the desired frequency due to noise in the laboratory environment. To combat these fluctuations and drifts, a variety of methods, such as frequency combs or optical cavities, are employed. However, these can be costly and/or more time-consuming to employ. Consequently, the use of atomic or molecular vapor cells provides stable references in compact and economical packages. The purpose of this project is to probe the […]
Alejandro Sanchez Ocegueda
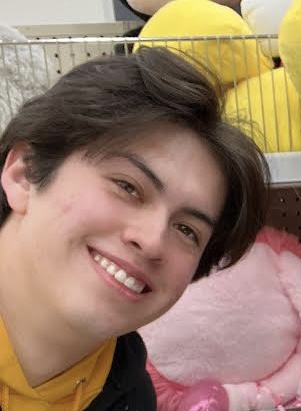
This summer, I will be using math to take down human trafficking rings, prevent the spread of diseases, and make companies more resistant against cyberattacks! You may be surprised, but it turns out that since criminal organizations, cities, and computer systems can all be modeled as networks, all these scenarios are actually the same problem in disguise! Indeed, in a branch of mathematics called graph theory, this problem is called “network dismantling.” In essence, dismantling a network boils down to removing enough components such that the network is broken up […]
Fangyuan Lin
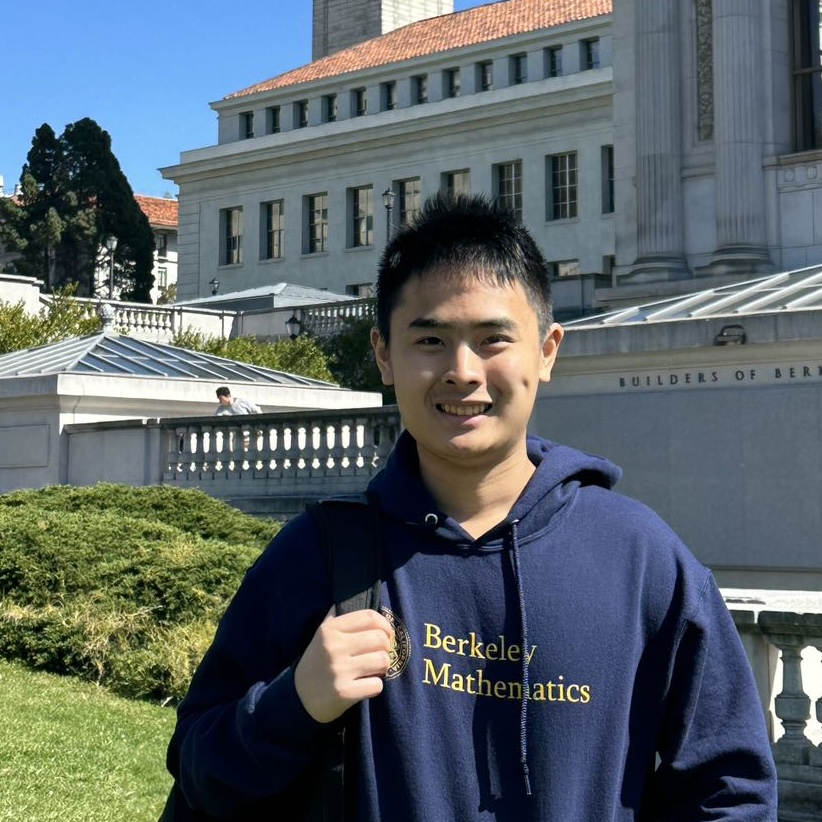
“A fundamental question that continually reappears in the study of Markov processes is, “When is a function of a Markov process also a Markov process?” A classical and somewhat obvious criterion was supplied by (Dynkin, 1965). Dynkin’s result gives a sufficient condition for such a state of affairs to hold for an arbitrary starting state. A much more subtle and general criterion involving the novel notion of intertwining of kernels was presented in (Rogers & Pitman, 1981). A key observation of this paper is that Markovianity may only hold for […]