Shiladitya Dutta
Stability Driven Interpretation of Low-rank Compression Methodologies for CNNs
Deep neural networks achieve state-of-the-art performance in many tasks such as computer vision and natural language processing, however many compression/acceleration methods for these models yield unstable or unreliable results. As such, I am using statistical methodologies to investigate the properties of low-rank compression methodologies, with a particular focus on SVD-based compression. In particular, I am focusing on how SVD compression can be used in compression with various filter-level visualization methods (i.e. saliency based methods) in order to reveal the internal “decision-making” structure of particular sections of these highly complex models. This will hopefully yield insights into how these compression methods could be used for the visualizations and interpretation of Neural Networks, something that is particularly relevant since utilizing “Black Box” algorithms poses a significant ethical dilemma in fields like medicine.
Message To Sponsor
I'd like to thank the donors of URAP for helping to sponsor this program. URAP has helped me, along with I'm sure many others, deepen my appreciation for research as an activity and as a career. By working on this program full time, I have learned many generally useful skills regarding how to work on intensive projects over time along with allowing me to deepen my knowledge within the subject matter of my project. As such, for this experience I sincerely thank you.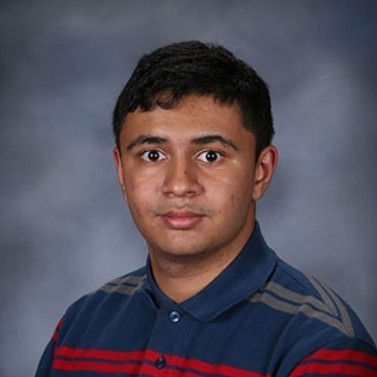