Yijin Wang L&S Math & Physical Sciences
Adaptive Strategies for PINNs: Addressing Spectral Bias in Fluids
This project aims to enhance the performance of Physics-Informed Neural Networks (PINNs) in simulating fluid dynamics, specifically addressing the challenge of spectral bias which can skew simulation accuracy. Our approach involves developing adaptive training strategies that empower PINNs to more precisely model a diverse range of fluid behaviors, from steady, predictable patterns to dynamic, turbulent flows. By integrating principles from the neural tangent kernel theory, we are meticulously fine-tuning the training processes of these networks. This involves recalibrating how PINNs learn from data, focusing on a balanced assimilation of both low- and high-frequency phenomena. The primary goal is to improve the precision of these simulations, thereby enhancing their application in scientific and engineering contexts. This refinement could lead to more reliable predictive models, supporting advanced research and practical applications in fluid dynamics.
Message To Sponsor
Dear Leadership Sponsor, I am deeply grateful for the opportunity to pursue my research on Physics-Informed Neural Networks (PINNs) and fluid dynamics through your generous support. This fellowship has not only deepened my understanding of these advanced concepts but also fueled my passion for scientific exploration and innovation. Your contribution has played a crucial role in my academic growth, empowering me to push the boundaries of my research and set the stage for future achievements. Thank you for your invaluable support in my journey. Sincerely, Yijin Wang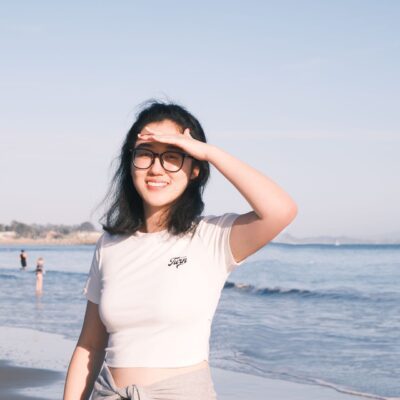