Noah Stevenson L&S Sciences
Tracking Non-Markovian Quantum Dynamics of a Superconducting Qubit via Deep Learning Filters
Theoretical and experimental evidence suggests harnessing quantum mechanics to execute algorithms on qubit-based quantum hardware may allow for computation exponentially more powerful than is possible with classical computers. Characterizing how qubit states evolve in time is imperative for benchmarking quantum hardware; however, this has been difficult due to the inability to fully measure a quantum state without disrupting it. A solution is weak measurement, which recent work has improved for a single qubit by leveraging the data-processing power of a recurrent neural network (RNN). The time-evolution of qubits exchanging information with their environment over long time scales is not well understood, but must be characterized to implement efficient algorithms on a quantum processor. I propose to use weak measurement and deep learning models as filters to characterize the time-evolution of a superconducting qubit exchanging information with a simplified environment. My project will contribute to the fields ongoing research in determining the time evolution of qubits and assess a technique for improving measurement accuracy of quantum systems.
Message To Sponsor
I would like to thank the Kay & Shaw fund with appreciation and gratitude for supporting me in pursuing research over the summer. Your support allowed me to contribute to our understanding of quantum devices, measurement and control, and collaborate with the leading experts in theoretical and experimental continuous quantum measurement. I feel privileged to have spent my summer months working with a such a skilled and experienced group of people on a compelling project. Aside from developing my physical understanding and technical know-how, this summer was particularly important in helping me reflect on my long-term academic goals and what questions I find interesting and valuable. After having overcome challenges I initially found baleful and realizing how much I've learned since the outset of my project, I leave the summer with increased confidence and assurance in my ability as a researcher, and a feeling of gratitude towards you for supporting me. Again, thank you.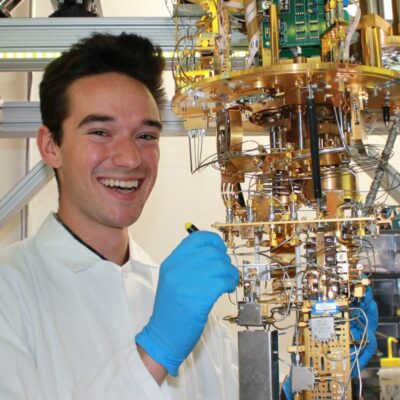