Lawrence Chen L&S Social Sciences
Exploration vs. Exploitation in Crowdsourcing Task Selection
Crowdsourcing platforms such as Amazon Mechanical Turk (MTurk) and Prolific are unique sources of income for workers preferring a flexible work arrangement and a low barrier to entry. While crowdsourcing research has primarily focused on optimizing worker performance from the manager’s perspective, this project shifts focus to how workers may strategically select tasks by balancing exploration versus exploitation to maximize income. The theoretical component of this project will utilize recent developments in multi-armed bandit problems to construct a novel mathematical model of crowdsourcing work from the worker’s perspective. The empirical component will employ a dataset of over 3 million completed MTurk tasks which I will use to test the model’s predictions via statistical methods. Insights gathered will highlight how workers adapt to task selection systems and why deviations from optimal task selection behavior may occur, offering important implications for the design of fairer and more efficient crowdsourcing platforms.
Message To Sponsor
Thank you for your support on this SURF project! This summer feels like a great opportunity to focus on my research without the academic and financial constraints of a regular semester, for which I am very grateful for. I hope to use my time to gain further domain expertise and to develop my research skills for graduate school success.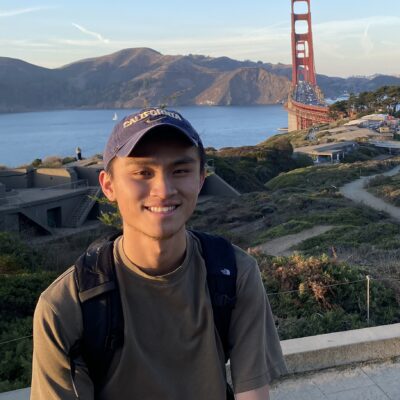