Jorge Diaz Chao L&S Math & Physical Sciences
AR Training Powered by Symbolic Learning and Large Language Models
We are building a novel platform that introduces a new dimension to asynchronous learning—like YouTube did upon launch—by empowering users with no coding experience to democratize their knowledge on tasks involving coordinated motor skills through Augmented Reality (AR) scenarios. Our work involves designing a user interface capable of inferring motor and linguistic cues from demonstrations and explanations, and writing an algorithm that synthesizes probabilistic code, namely Scenic, that models motion primitives to build complex behaviors. To do so, we employ tools such as symbolic learning and Large Language Models (LLMs) as opposed to relying on other conventional methods like Deep Neural Networks (DNNs) to solve popular challenges in the field of Human-Robot Interaction (HRI), where data is sparse. Ultimately, users will have access to expert knowledge through simulated scenarios that replicate the stochastic nature of the physical world. The algorithm will dynamically adapt to contextual nuances, providing tailored instructions based on the expert’s demonstration.
Message To Sponsor
Your contributions are important to support the passion of many students like me to experiment with research and build future technologies. Many are the students that are discouraged by research due to the lack of funding or opportunities, but programs like SURF inspire students to keep improving on technology and solving global issues.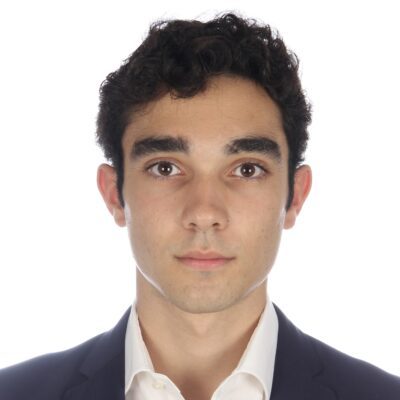