Jingyuan Chen L&S Math & Physical Sciences
Graphons and Neural Networks
Neural networks have become ubiquitous in recent years, however, their theoretical underpinnings remain limited – there is a wide gap between practical performance and rigorous understanding.
An object created in part by CDSS Dean Chayes called the Graphon offers a new perspective for this challenge. A Graphon is a continuous function that represents the limit of an infinitely large, dense graph, essentially a “”continuous adjacency matrix”” for a network. Existing literature exists in modelling GNN outputs when sampling from a graphon, but not much work has been done on modelling the internal architectures of neural networks with dense graphs. While Graphons originally live in the dense regime, whereas NN are somewhat sparse, there have been development in both fields with the creation of sparse Graphons as well as dense regimes in neural networks (eg. fully connected layers).
This research aims to expand the application of graph theory to include the internal structure of neural networks through objects like the graphon. These insights could lead to more principled network designs, improved model pruning strategies, and better guarantees for network expressivity and stability.
Message To Sponsor
Thank you so much for sponsoring my research project! As an international student on a budget, your contribution has allowed me to explore a topic that is typically not introduced in the undergraduate curriculum yet can have great contributions towards many fields of research.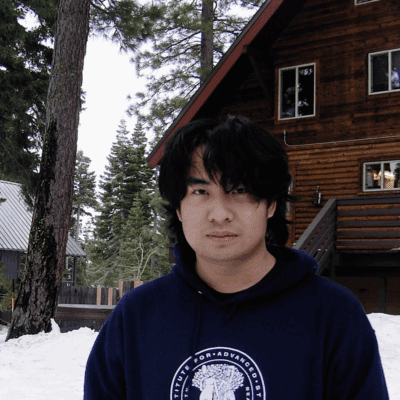