Ryan Lehmkuhl Rose Hills
Secure Prediction for Neural Networks
Machine learning classification is growing increasingly important for a variety of industries and applications, including medical imaging, spam detection, facial recognition, financial predictions, and more. As understanding of these systems advances, so do attacks which seek to exfiltrate information from exposed models. These models are often trained on confidential data and leakscan compromise user privacy.
Additionally, users may wish to receive classifications on a modelwhile keeping their own input secret from the service provider. To address these concerns, I introduce the concept of secure prediction. Secure prediction defines a joint computation between the user and service provider where the user receives the classification of their information on the providers model, but neither side learns anything about each others input. Generally speaking, secure prediction protocols incur huge penalties in either computation, bandwidth, or latency compared to traditional prediction. My work combines several techniques in a novel protocol which cleverly manages these blowups in order to construct a realistic system.
Message To Sponsor
To the Rose Hills Foundation: Thank you so much for giving me the opportunity to do research!! I've done internships for the last 3 summers, and this was definitely the most rewarding work I've ever been able to do. Having the opportunity to do research all summer has convinced me to apply for grad school for my PhD - something I was barely considering before now.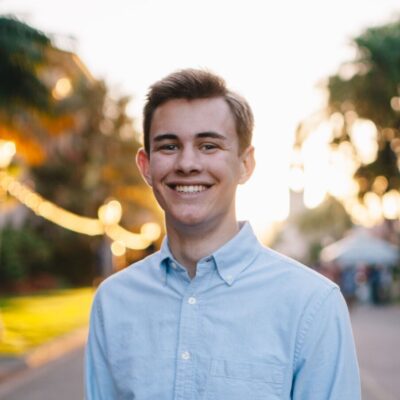