Levi Galvan L&S Math & Physical Sciences
Physics-Informed Neural Networks (PINN) for Exoplanet Detection
This project aims to create a physics-informed convolutional neural network (CNN) to improve the detection and characterization of Earth-like exoplanets from stellar light curves, especially in the low signal-to-noise regime. Traditional CNNs have shown promising performance in classifying transit signals but often function as “black boxes,” relying solely on data-driven features and ignoring key astrophysical constraints. To address this, my approach embeds analytical transit models and physical priors such as limb-darkening, transit symmetry, and realistic depth-duration relationships directly into the network’s architecture or loss function. These constraints will guide the model toward physically plausible outputs and reduce false positives. The model will be trained on real light curves from Kepler and TESS, with synthetic transits injected to evaluate recovery rates under noisy conditions. While a full detection pipeline is most likely beyond the scope of the summer, this work will lay the foundation for a more interpretable and robust detection method.
Message To Sponsor
I am very grateful for the opportunity to pursue my research through your generous support. This project combines my passions for astrophysics and machine learning to explore new ways of detecting Earth-like exoplanets using physics-informed neural networks. Your contribution allows me to investigate meaningful questions at the intersection of theory and data, and take an important step toward my long-term goal of advancing exoplanet science. Thank you for helping make this work possible.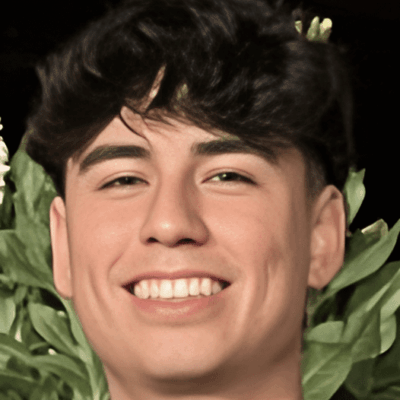